In a world where AI promises incredible problem-solving powers, instant global communication, and information exchange, recent news stories serve as a sobering reminder of the technology’s cultural blind spots and biases— and the potential for cultural bias to persist when content is translated.
However, even though AI translation is fast and cost-effective, it can trip over cultural nuances and introduce various forms of bias, creating problems in clinical trials, patient health, compliance, and other healthcare settings. Here are 2 examples of cultural bias in AI related to healthcare:
- AI systems are more likely to attribute symptoms reported by patients from non-Western cultures as being related to traditional beliefs or practices, rather than considering medical explanations. This bias could lead to cultural stereotypes influencing medical decisions and marginalizing patients’ concerns.
- An AI system is trained on a dataset of medical records that disproportionately represents certain demographic groups and may perpetuate stereotypes or biases about those groups. Conversely, an AI system trained on data that excludes a certain demographic group will fail to consider factors related to that group in its translations.
Farhanna Sayegh, Executive Director of Multicultural Marketing at CQ fluency says, “These examples and others like them are a wake-up call for organizations relying on AI to reach multilingual consumers, especially for life sciences and healthcare businesses where bias and errors can harm people.”
As businesses lean more into AI for global communication needs, it’s imperative to recognize and address the potential problems AI systems may introduce when generating information or translations for use in healthcare settings.
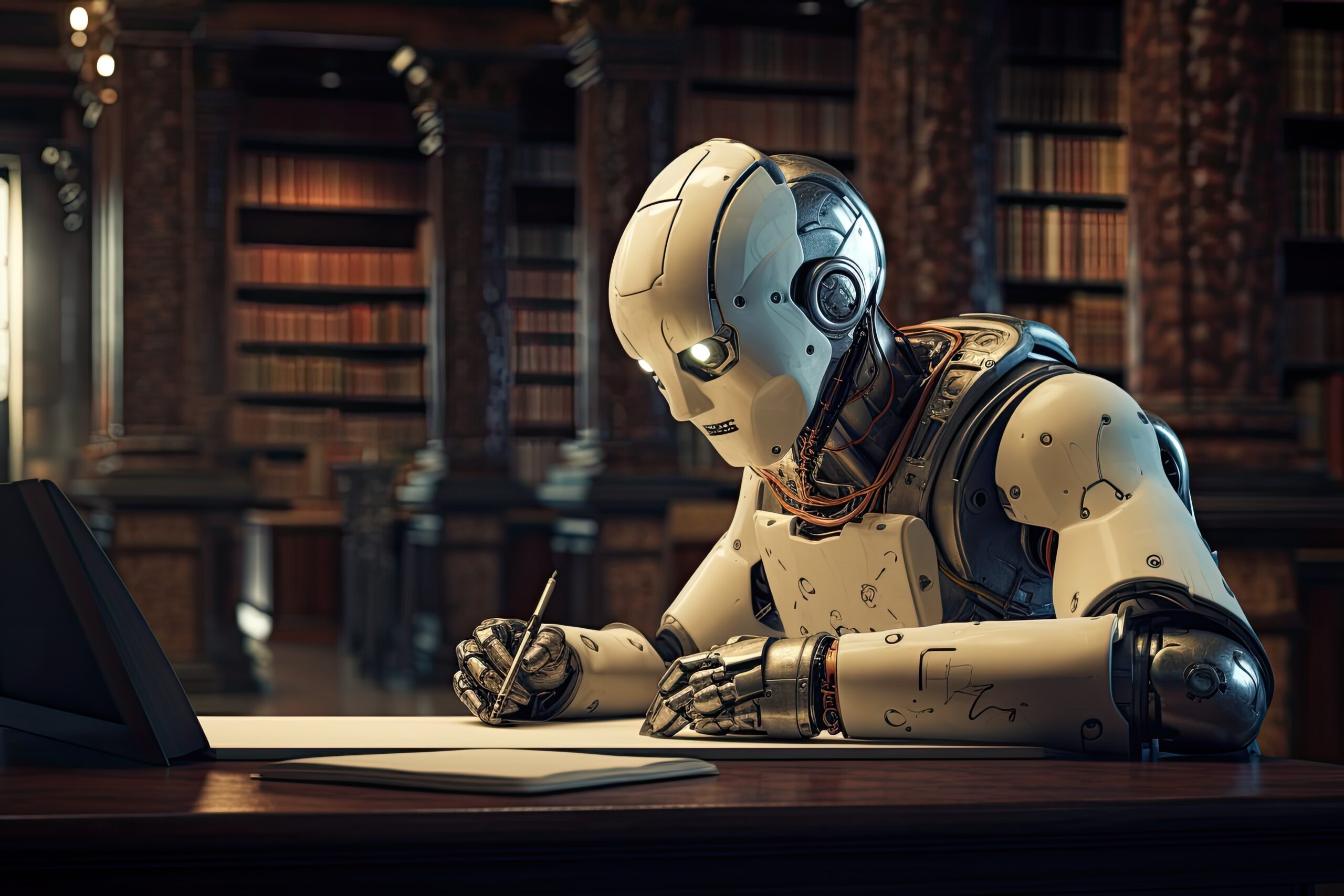
what does bias look like in AI translations?
As AI translation gets better, research shows that bias is actually worsening. A study in Cambridge University Press states, ‘Given that language use is heavily influenced by the culture of the host country and carries with it deeply ingrained perceptions, beliefs, and attitudes, increasingly fluent translations can increasingly convey those cultural aspects, and sometimes bring cultural biases along with them. In this respect, machine biases induced by translation are inevitable.’
Let’s explore some of the different types of bias in AI along with their potential consequences and causes. As organizations move toward using generative AI to create content for a multilingual, multicultural audience, it’s important to be aware of and correct for these tendencies.
gender bias
AI translation systems have been shown to stereotype emotions, classifying words like “anxious” and “depressed” as feminine. When a language model thinks that these adjectives align with the female gender, even though it’s not true, then it bias could lead to health concerns being dismissed or misdiagnosed.
Also, when translating from a gender-neutral language like English to a gender-specific one like Spanish, AI often has to guess genders. If there’s no pronoun to indicate whether the noun in question is male or female, it will pick the option that is statistically more likely based on its training data.
As a result, if there’s any ambiguity, stereotypically male roles like “doctor” tend to be translated as male. Stereotypically female professions like “nurse” or “house cleaner” will be given feminine forms. In both cases, the choice made by the algorithm reinforces these outdated stereotypes.
Lastly, when the source language uses non-inclusive gender-specific terms, like ‘man-made’ or ‘chairman’ in English, those terms are simply translated to the equivalent in the new language by AI, preserving that bias that was in the source language.
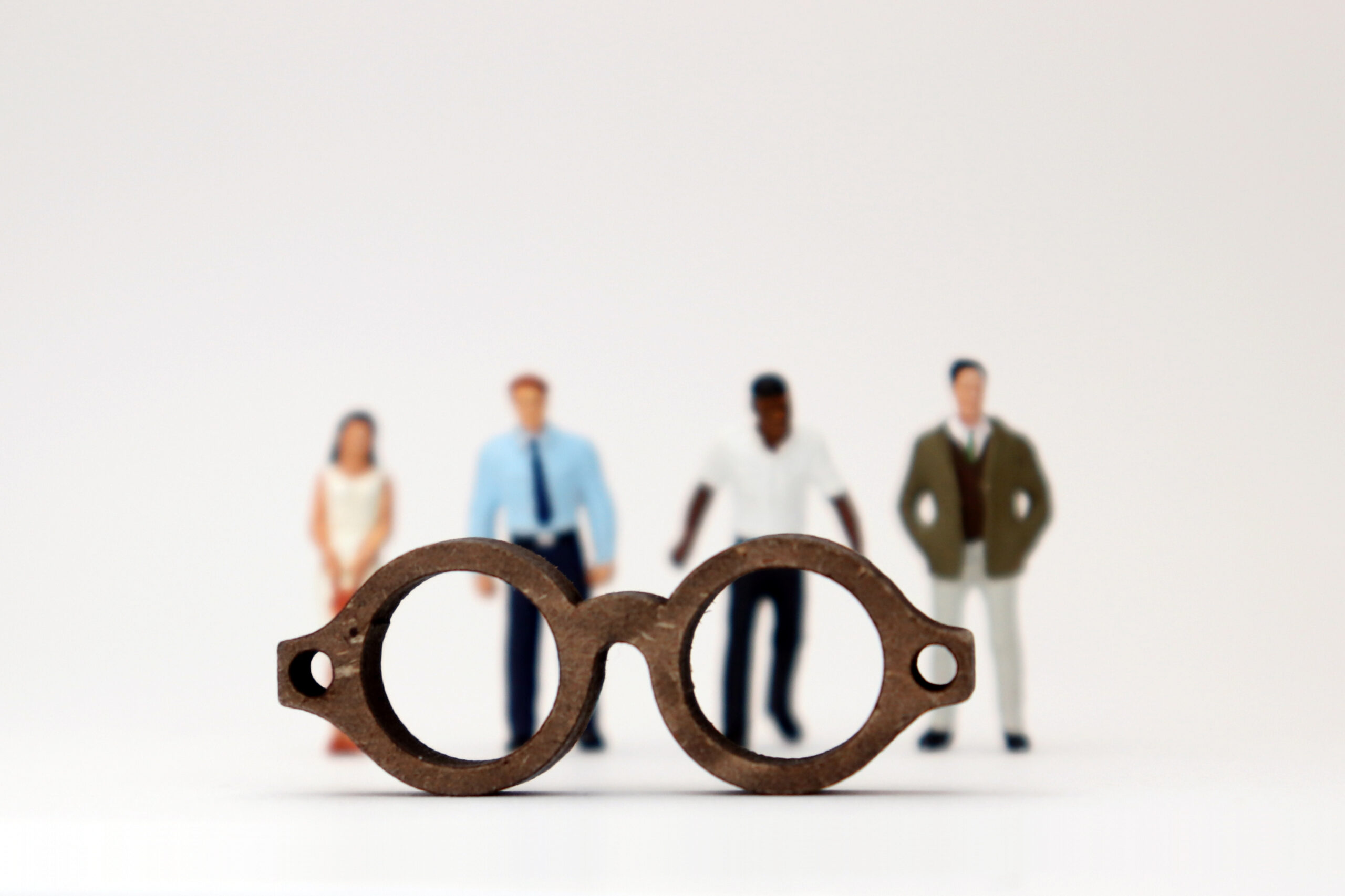
racial bias
In June of this year, an analysis of more than 5,000 images generated by Stability AI found that the program generally showed people with lighter skin tones holding more prestigious jobs, while people with darker skin tones were represented holding low-paying jobs like dishwashers and housekeepers.
Also, content translated by AI is likely to represent symptoms reported by Black patients as being related to pain or drug-seeking behavior, while symptoms reported by white patients were more likely to be translated as being related to medical conditions. This bias could lead to Black patients receiving less comprehensive or appropriate care.
Consider what would happen if AI were to generate patient descriptions in a medical research article without correcting for bias. If it skews towards lighter skin tones for prestigious roles, it’s not just unfair – it’s a misrepresentation that could affect patient trust and clinical trial recruitment.
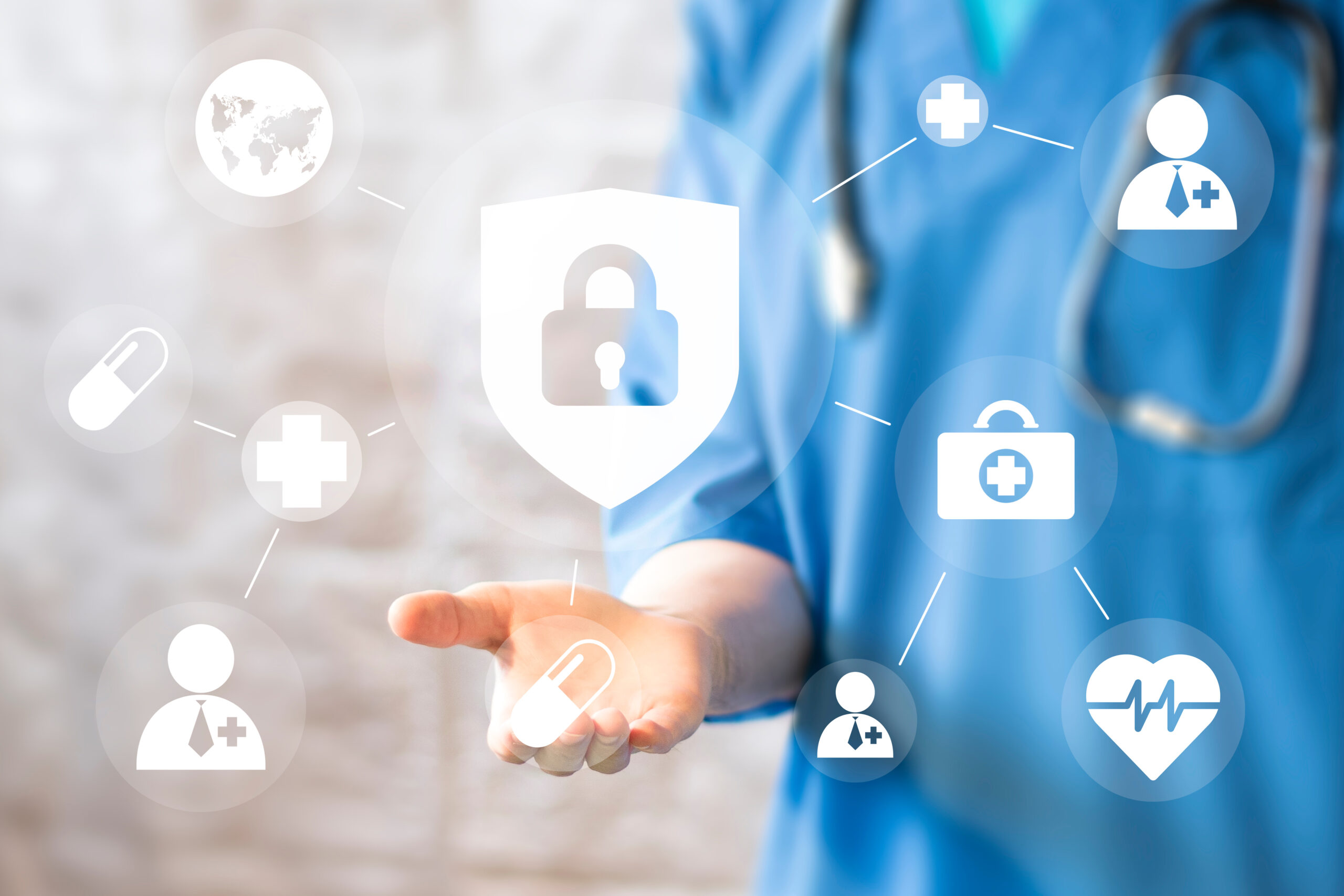
cultural bias
Different cultures practice different customs, traditions, and holidays. They have different family dynamics and religious beliefs. Food and dietary restrictions also vary between cultures, as do views on different types of illness, especially mental illness.
If AI translations don’t consider these cultural differences, patients might end up with advice or information that doesn’t fit their lifestyle or beliefs. For example, in most Westernized countries, counseling is widely seen as an effective therapy for mental illnesses, especially where trauma is involved. However, talking about painful events may not be seen as valuable by people from societies with different cultural preferences and practices. In these cases, other options like movement-based therapies or expressive therapies might be better options, and translated content needs to reflect these differences.
Studies also show that AI can associate Muslims with words like terrorism and Mexicans with poverty. These incorrect stereotypes can affect caregiver perception and interfere with high-quality care.
Cultural bias is a persistent problem in healthcare and life sciences as a whole, and AI has the potential to reinforce it if it’s used without the proper process, tools, and expert guidance in place.
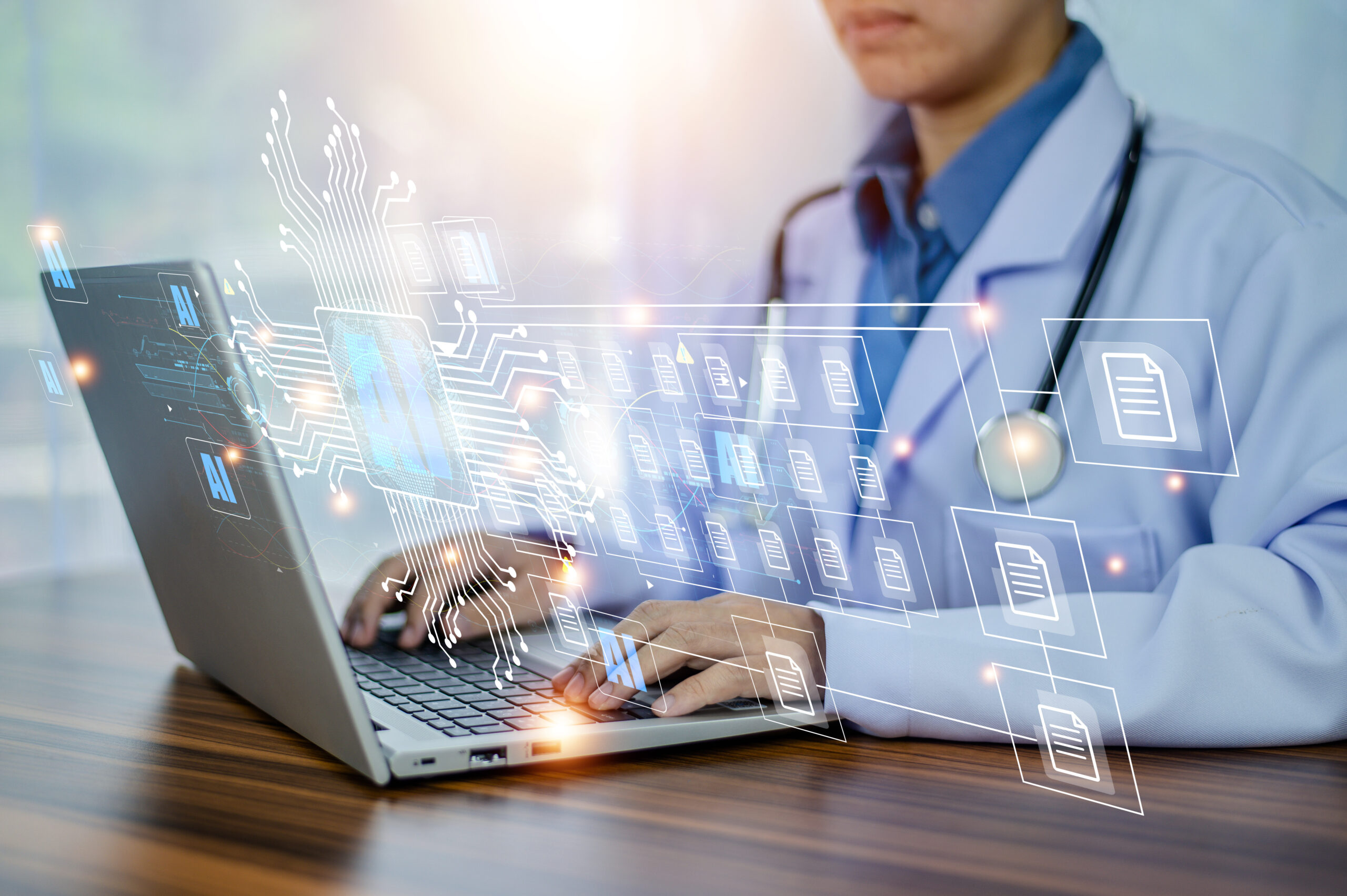
how training data bias corrupts AI translation models
AI systems are commonly thought of as neutral or impartial (because they aren’t human), but that is not the case. Large Language Models (LLMs) like ChatGPT are trained on vast amounts of public data, and much of that data contains stereotypes, biases, and prejudices. Also if data is more than a few years old, it likely doesn’t reflect recent efforts in society to be more inclusive.
Sayegh notes that “Huge datasets are required to train AI translation engines. That data is full of bias, especially related to cultural differences, and it’s carried over into translations in damaging ways.”
Problems in training data can also include:
- “sampling bias” in which some groups are overrepresented or underrepresented in the data
- “labeling bias” when humans labeling the data perpetuate their own bias when they perform the task of labeling or classifying data
- “algorithmic discrimination” in which bias is embedded in the optimization processes applied during training. In this example, the software itself is biased.
- “contextual bias” – lack of understanding of the broader social and cultural contexts
- “biased feedback loops” or “self-reinforcing bias” or a “cyclical bias” which are reinforced when an AI system is deployed in a real-world setting and its decisions impact subsequent data collection.
clearly, the data is dirty
Using what it’s given, AI learns from and reflects these prejudices, resulting in outputs and translation errors that can be culturally insensitive, spread harmful stereotypes, and create potential hazards, especially in a life science setting where accurate information is everything.
So, why has this problem with bias in the data not been fixed yet? In part because there is simply so much data to fix.
The data to be used for training is cleansed and prepared when deploying AI translation methodologies like Neural Machine Translation (NMT). However, in many cases, the data isn’t fully reviewed and cleaned because it’s expensive and time-consuming to do so. In other cases, important data may be missing.
Data that is not audited and cleaned of bias will automatically and naturally generate content that brings the bias forward—unless we can impose some form of control.
It’s also important to note that a frequent lack of diversity in development teams can bring in bias. While many companies are working hard towards diversity in their staff, most organizations aren’t there yet. At CQ fluency we are proud of the diversity of our team overseeing these programs/projects.
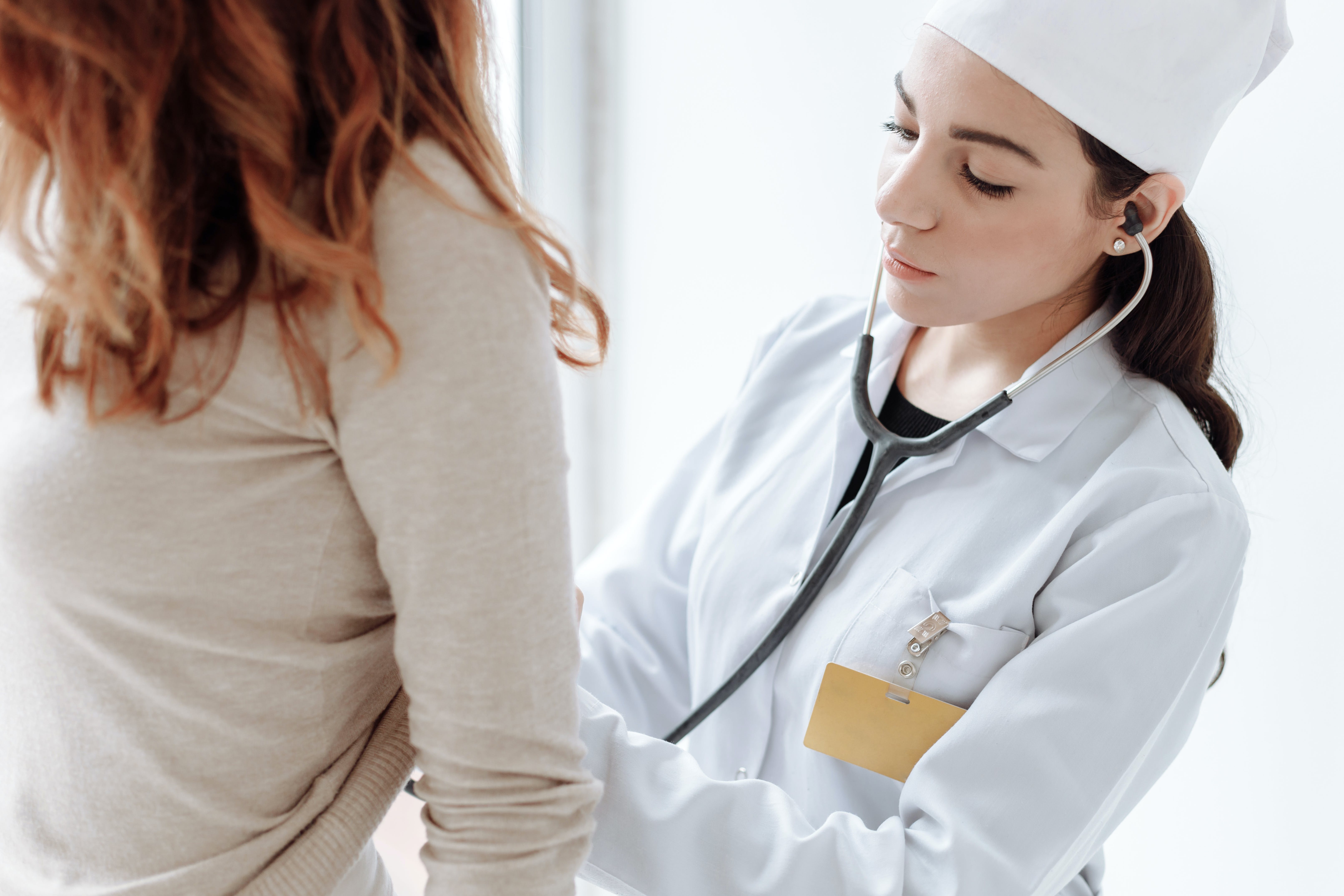
the path forward
AI-powered translations are appealing for brands that need to tackle high volumes, but it’s hard (and ill-advised) for businesses to go all in when we see that the outcome inherits damaging biases from its data and creators. There are human, training data, algorithmic, and even language biases at play. This mix can yield problematic results, a concern especially for healthcare organizations.From the Cambridge University study: ‘Since human thoughts and behaviors do have social and cultural contexts, sexist, racial, class, and other types of bias are inevitable in MT output; and artificial intelligence, as our brainchild, will inevitably amplify these tendencies. Nevertheless, they are predictable and preventable.’
Businesses cannot assume it is safe to use Machine Translated content as is.
The fix? Human oversight. Trained linguists must post-edit AI translations, finding and fixing bia Retraining data sets with the revised translations + data annotation/tagging to help AI adapt results based on cultural / race / gender. Continues to improve results each time making human oversight / fixes less and less as time goes on.ses and inaccuracies. However, this fully manual QA process can be slow and pricey. Then, engines must be retrained with revised translations. This process will continue to improve results each time, reducing the need for human oversight and manual fixes over time.
To unlock the speed of AI and solve the problem of bias, businesses will need a three-pronged approach: human expertise, automated quality checks, and custom-designed tools. This combo will help us achieve accurate, unbiased translations at scale.
At CQ fluency, we’re committed to fighting bias. We’re building protocols, processes, and tools to ensure translations reflect target cultures’ values and nuances and are free from any kind of bias.
We’ll show you how we do this in the next post in our series “More Culture Relevance, Less Bias: Optimizing AI-powered Translations”. Stay tuned for more information on how your life sciences or healthcare organization can use AI to its full potential.